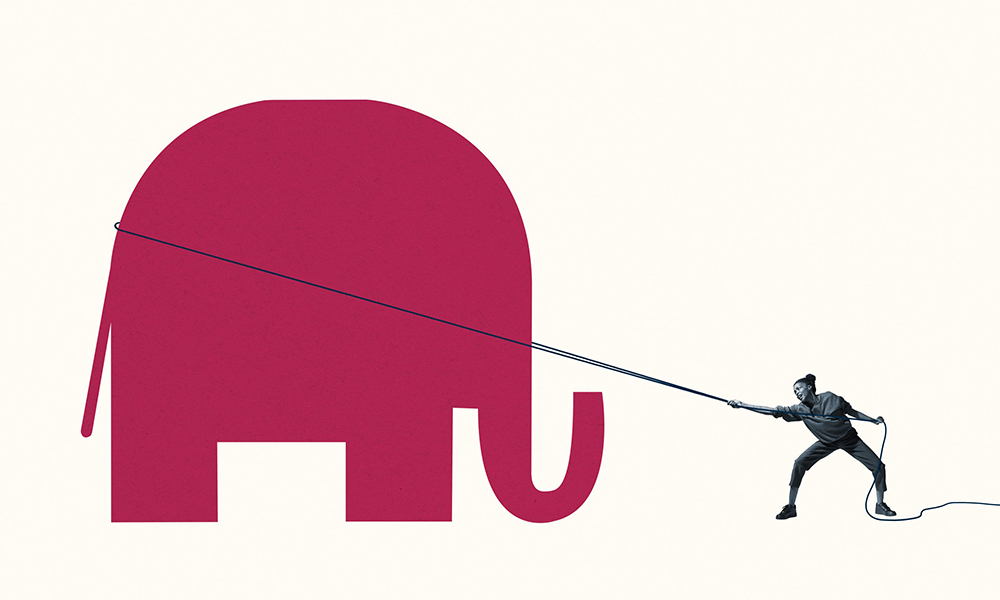
尽管生成式人工智能近年来取得了显著进步,但其应用仍然主要局限于那些历来在部署新兴技术上占据先机的大型企业。但随着生成式人工智能技术的持续演进,最适合从中获取价值的企业类型也在不断变化。越来越多的中型企业凭借在资源和敏捷性方面取得的巧妙平衡,正加速推进生成式人工智能的应用步伐,推动产生有意义的成果,并在技术成熟的过程中从中获益。
大型企业目前在生成式人工智能的采用方面占据主导地位。波士顿咨询公司(BCG)2024年的一项调查发现,46%的大型企业报告称其在生成式人工智能成熟度方面处于中等至较高水平,而中型企业的这一比例仅为28%。得益于在人才招募、数据获取以及资金筹备方面的便利条件,规模较大的企业在传统资源密集型人工智能项目中更具优势。
但这种优势似乎正在减弱。伦敦商学院(LBS)、Institute of Directors和Evolution Ltd最近的研究表明,中型企业,尤其是那些雄心勃勃、专注于增长的企业,比如那些获得私募股权支持的企业,在克服采用障碍方面处于有利地位。与大型企业相比,中型企业更加灵活,这成为推动生成式人工智能日益普及的一个关键因素,也使得这些企业成为利用该技术并释放其潜力的理想候选者。
总体而言,虽然这些公司仍处于落后状态,但它们似乎已蓄势待发,准备迎头赶上。牛津经济研究院(Oxford Economics)的研究发现,在接受调查的中型企业中,只有四分之一在2023年采用了人工智能,但51%的企业计划在2024年采用人工智能;采用该技术的企业期望人工智能能改善它们的前景,特别是在新产品和服务(43%)以及营销和销售(48%)方面。
组织规模:一把双刃剑
直到最近,从生成式人工智能中获益最多的还是规模(非常)大的公司,因为规模优势足以克服因规模扩大而带来的组织复杂性挑战。然而,随着技术的发展,大型企业发现自己难以迅速适应。多层级的管理架构、根深蒂固的流程和部门间的隔阂会减缓像生成式人工智能这样快速发展的技术的采用速度。
在大型企业中,生成式人工智能的实施可能会遭遇“千个项目试点失败”,即各个团队或职能部门开发概念验证产品和工具,但由于企业复杂性和缺乏明确的治理,无法将其规模化。因此,尽管在数字化转型方面投入巨大,大型企业往往难以充分发挥新工具的潜力。
相比之下,中型企业则可以从更精简的组织结构中受益,从而实现更为迅速的决策和执行,前提是具备恰当的领导力和治理机制。当它们的敏捷性与正确的战略相结合时,就能更迅速地适应技术领域的新动态,并且更轻松地将生成式人工智能投入运营。(这里所说的中型企业指的是收入在5000万美元到10亿美元之间的公司,尽管具体的定义因国家而异,但中型企业广义上指的是规模相对较小、运营相对简单且能保持敏捷性的企业。)
虽然体量和规模优势曾在获得专业人才及构建资本密集型基础设施方面发挥了决定性优势,但生成式人工智能技术的发展,尤其是作为服务的生成式人工智能的发展、精简平台的出现以及可定制模型的增长,正在为中型企业和大型企业创造一个更加公平的竞争环境。
例如,生成式人工智能供应商通过提供模型和基础设施即服务,极大地降低了对前期投资和广泛信息技术能力的需求。像谷歌Vertex AI和Snowflake这样的精简平台解决方案也简化了人工智能生态系统,提供集成化的数据管理、模型定制和部署工具,所有这些都降低了技术门槛,并加速了价值实现的时间进程。
与此同时,通过检索增强生成(RAG)等技术实现的可定制模型的进步,使中型企业能够有效地利用其专有数据,而无需拥有一支庞大的内部数据科学家团队。构建传统人工智能所需的大部分编码工作已被自然语言提示工程所取代,从而能够创建适合公司内容、专业知识和工作流程的生成式人工智能驱动工具。
此外,包括企业资源计划(ERP)和客户关系管理系统(CRM)在内的现有软件平台的更新融入了人工智能功能,从而可以轻松访问现有技术堆栈上的人工智能功能。鉴于中型企业通常拥有复杂程度较低且定制化程度不高的软件实例,因此它们能够比大型企业更快、更轻松地集成这些新功能。
从采用生成式人工智能到创造价值
除了采用生成式人工智能之外,中型企业在利用其创造价值方面也占据了得天独厚的优势,因为这可能助力它们突破那些常常制约其发展的运营限制。中型企业往往难以吸引数据科学家等专业人才,而且规模也不足以使其聘请全职人员变得经济可行。生成式人工智能工具能够拓展现有员工的能力。正如波士顿咨询公司最近的一项实验所证明的那样,在该实验中,每位管理顾问都被要求完成三项超出其核心咨询能力的基本数据科学任务:数据清理、预测分析和统计理解。
使用生成式人工智能来执行任务,顾问们的能力立即得到了提升,超出了他们现有的能力范围。相较于未采用生成式人工智能的参与者,这些借助了生成式人工智能的参与者的能力提高了13到49个百分点,与数据科学家的基准水平相差12到17个百分点。针对特定职能或角色的工具正在进入市场,使公司能够进一步扩展现有员工的能力。例如,Sisense使公司无需编写代码即可构建语义数据模型,用户可以通过自然语言查询进行查询,从而使管理人员能够将数据驱动的洞察纳入决策,而无需数据分析师或数据科学家的协助。
在规模较小的企业中,另一个常见的限制因素是缺乏足够的专有数据来实现差异化。伦敦商学院、Institute of Directors和Evolution Ltd.近期开展的一项研究发现,年收入在1000万至5000万英镑之间的小型公司中,仅有56%的公司表示专有知识对其业务具有一定的乃至至关重要的作用,而在年收入超过5000万英镑的中型公司中,这一比例为72%。相比之下,大型企业早已利用传统的人工智能从专有数据中挖掘价值,并且在数据清理和整合方面进行了投资。
然而,中型企业通常拥有大量非结构化数据,却难以从中提取价值。例如,一家中型企业可能会为客服代表配备了详尽的产品说明手册与故障排查指南,以及真实客户支持电话的通话记录。借助生成式人工智能,这类企业如今无需聘请数据科学家团队,就能利用公司数据建立新关联,并实时创建和传播高度定制化的组织知识,从而释放洞察力。其结果是企业能够以更低的成本改善客户服务——这是这些企业过去因资源、能力和基础设施局限而无法做到的。
由私募股权公司支持的中型企业在运营层面拥有额外的优势——战略协同、财务和人力资本以及专注的执行力——这使它们成为采用生成式人工智能的首选对象。私募股权公司为其投资组合公司设定了明确的目标和时间表,专注于在特定投资期限(通常为五年)内创造价值,这使得它们能够果断采取行动,优先考虑并实施生成式人工智能应用。获得私募股权支持的公司还可以获得生成式人工智能项目所需的财务和人力资本,使这些公司能够在预期增长的情况下大力投资于领导团队和顾问团队建设。因此,这些企业往往更愿意基于高回报的潜力而承担经过深思熟虑的风险。
中型企业利用生成式人工智能的策略
相较于大型企业,中型企业在采用生成式人工智能方面可能有一些结构性优势,但这并不能保证成功。以下列举了当前中型企业可采取的五大战略举措,旨在提升其成功运用生成式人工智能并实现价值创造的可能性。
1. 构建可扩展且灵活的生成式人工智能技术栈:投资可扩展的人工智能即服务平台,这些平台能够随着公司的发展而扩展,而无需大量额外投资。
2.转向“重塑”和“发明”: 除了部署生成式人工智能来逐步改进现有流程外,还要重新思考业务模式以及如何重新设计整个功能。波士顿咨询公司最近的一项调查发现,处于人工智能应用前沿的公司在核心业务功能中部署人工智能和生成式人工智能所获得的价值占比高达近三分之二(62%),其余三分之一(38%)则来自更多的外围支持功能。由此得出的启示显而易见:选择能够重新设计核心功能的深层应用程序,并优先考虑那些利用独特、专有数据来创建护城河的应用程序。
3. 关注能与生成式人工智能相辅相成的因素,而不仅仅是技术本身:正如Evolution Ltd最近发布的一份白皮书所指出的那样,人们对生成式人工智能感到失望的一大关键原因在于过于强调技术本身,而对上游(数据工程和专有数据)和下游(将生成式人工智能整合到战略决策中并创建学习和实验循环)的关注太少。
4. 建立清晰的治理和领导机制:要在生成式人工智能方面取得成功,公司领导层必须做出坚定承诺,构建有利于高效决策的治理结构,并优先考虑中期投资,而不仅仅是即时回报。
5. 增强员工能力:利用生成式人工智能增强员工技能,使他们能够完成超出当前能力范围的任务。
生成式人工智能:中型企业的“最佳时机”?
中型企业过去被认为规模太小,如今可能“恰好”适合充分利用当今的生成式人工智能。然而,要把握住这一机遇,它们需要制定明确的战略,并密切关注生成式人工智能能够发挥作用的领域——不只是降低成本,还要创造收入和价值。那些能够始终专注于有效推进生成式人工智能战略实施的企业会发现,人工智能革命并非只是行业巨头或灵活的初创企业的专属,而是包容性的变革浪潮,中型企业是搭乘“革命专列”的理想之选。(财富中文网)
弗朗索瓦·坎德隆(François Candelon)是私募股权公司Seven2的合伙人,曾任波士顿咨询公司亨德森研究所(BCG Henderson Institute)全球主管。
迈克尔·G·雅各比德斯(Michael G. Jacobides)是伦敦商学院唐纳德·戈登爵士创业与创新教授,同时担任波士顿咨询公司亨德森研究所学术顾问和Evolution Ltd.首席顾问。
米纳尔·波尔(Meenal Pore)是波士顿咨询公司的负责人,同时也是波士顿咨询公司亨德森研究所的代表人物。
列昂尼德·茹科夫(Leonid Zhukov)现任波士顿咨询公司全球人工智能研究所所长,并兼任BCG.X人工智能与数据科学副总裁。
本专栏文章中所提及的部分公司是作者所在企业过去或现在的客户。
译者:中慧言-王芳
尽管生成式人工智能近年来取得了显著进步,但其应用仍然主要局限于那些历来在部署新兴技术上占据先机的大型企业。但随着生成式人工智能技术的持续演进,最适合从中获取价值的企业类型也在不断变化。越来越多的中型企业凭借在资源和敏捷性方面取得的巧妙平衡,正加速推进生成式人工智能的应用步伐,推动产生有意义的成果,并在技术成熟的过程中从中获益。
大型企业目前在生成式人工智能的采用方面占据主导地位。波士顿咨询公司(BCG)2024年的一项调查发现,46%的大型企业报告称其在生成式人工智能成熟度方面处于中等至较高水平,而中型企业的这一比例仅为28%。得益于在人才招募、数据获取以及资金筹备方面的便利条件,规模较大的企业在传统资源密集型人工智能项目中更具优势。
但这种优势似乎正在减弱。伦敦商学院(LBS)、Institute of Directors和Evolution Ltd最近的研究表明,中型企业,尤其是那些雄心勃勃、专注于增长的企业,比如那些获得私募股权支持的企业,在克服采用障碍方面处于有利地位。与大型企业相比,中型企业更加灵活,这成为推动生成式人工智能日益普及的一个关键因素,也使得这些企业成为利用该技术并释放其潜力的理想候选者。
总体而言,虽然这些公司仍处于落后状态,但它们似乎已蓄势待发,准备迎头赶上。牛津经济研究院(Oxford Economics)的研究发现,在接受调查的中型企业中,只有四分之一在2023年采用了人工智能,但51%的企业计划在2024年采用人工智能;采用该技术的企业期望人工智能能改善它们的前景,特别是在新产品和服务(43%)以及营销和销售(48%)方面。
组织规模:一把双刃剑
直到最近,从生成式人工智能中获益最多的还是规模(非常)大的公司,因为规模优势足以克服因规模扩大而带来的组织复杂性挑战。然而,随着技术的发展,大型企业发现自己难以迅速适应。多层级的管理架构、根深蒂固的流程和部门间的隔阂会减缓像生成式人工智能这样快速发展的技术的采用速度。
在大型企业中,生成式人工智能的实施可能会遭遇“千个项目试点失败”,即各个团队或职能部门开发概念验证产品和工具,但由于企业复杂性和缺乏明确的治理,无法将其规模化。因此,尽管在数字化转型方面投入巨大,大型企业往往难以充分发挥新工具的潜力。
相比之下,中型企业则可以从更精简的组织结构中受益,从而实现更为迅速的决策和执行,前提是具备恰当的领导力和治理机制。当它们的敏捷性与正确的战略相结合时,就能更迅速地适应技术领域的新动态,并且更轻松地将生成式人工智能投入运营。(这里所说的中型企业指的是收入在5000万美元到10亿美元之间的公司,尽管具体的定义因国家而异,但中型企业广义上指的是规模相对较小、运营相对简单且能保持敏捷性的企业。)
虽然体量和规模优势曾在获得专业人才及构建资本密集型基础设施方面发挥了决定性优势,但生成式人工智能技术的发展,尤其是作为服务的生成式人工智能的发展、精简平台的出现以及可定制模型的增长,正在为中型企业和大型企业创造一个更加公平的竞争环境。
例如,生成式人工智能供应商通过提供模型和基础设施即服务,极大地降低了对前期投资和广泛信息技术能力的需求。像谷歌Vertex AI和Snowflake这样的精简平台解决方案也简化了人工智能生态系统,提供集成化的数据管理、模型定制和部署工具,所有这些都降低了技术门槛,并加速了价值实现的时间进程。
与此同时,通过检索增强生成(RAG)等技术实现的可定制模型的进步,使中型企业能够有效地利用其专有数据,而无需拥有一支庞大的内部数据科学家团队。构建传统人工智能所需的大部分编码工作已被自然语言提示工程所取代,从而能够创建适合公司内容、专业知识和工作流程的生成式人工智能驱动工具。
此外,包括企业资源计划(ERP)和客户关系管理系统(CRM)在内的现有软件平台的更新融入了人工智能功能,从而可以轻松访问现有技术堆栈上的人工智能功能。鉴于中型企业通常拥有复杂程度较低且定制化程度不高的软件实例,因此它们能够比大型企业更快、更轻松地集成这些新功能。
从采用生成式人工智能到创造价值
除了采用生成式人工智能之外,中型企业在利用其创造价值方面也占据了得天独厚的优势,因为这可能助力它们突破那些常常制约其发展的运营限制。中型企业往往难以吸引数据科学家等专业人才,而且规模也不足以使其聘请全职人员变得经济可行。生成式人工智能工具能够拓展现有员工的能力。正如波士顿咨询公司最近的一项实验所证明的那样,在该实验中,每位管理顾问都被要求完成三项超出其核心咨询能力的基本数据科学任务:数据清理、预测分析和统计理解。
使用生成式人工智能来执行任务,顾问们的能力立即得到了提升,超出了他们现有的能力范围。相较于未采用生成式人工智能的参与者,这些借助了生成式人工智能的参与者的能力提高了13到49个百分点,与数据科学家的基准水平相差12到17个百分点。针对特定职能或角色的工具正在进入市场,使公司能够进一步扩展现有员工的能力。例如,Sisense使公司无需编写代码即可构建语义数据模型,用户可以通过自然语言查询进行查询,从而使管理人员能够将数据驱动的洞察纳入决策,而无需数据分析师或数据科学家的协助。
在规模较小的企业中,另一个常见的限制因素是缺乏足够的专有数据来实现差异化。伦敦商学院、Institute of Directors和Evolution Ltd.近期开展的一项研究发现,年收入在1000万至5000万英镑之间的小型公司中,仅有56%的公司表示专有知识对其业务具有一定的乃至至关重要的作用,而在年收入超过5000万英镑的中型公司中,这一比例为72%。相比之下,大型企业早已利用传统的人工智能从专有数据中挖掘价值,并且在数据清理和整合方面进行了投资。
然而,中型企业通常拥有大量非结构化数据,却难以从中提取价值。例如,一家中型企业可能会为客服代表配备了详尽的产品说明手册与故障排查指南,以及真实客户支持电话的通话记录。借助生成式人工智能,这类企业如今无需聘请数据科学家团队,就能利用公司数据建立新关联,并实时创建和传播高度定制化的组织知识,从而释放洞察力。其结果是企业能够以更低的成本改善客户服务——这是这些企业过去因资源、能力和基础设施局限而无法做到的。
由私募股权公司支持的中型企业在运营层面拥有额外的优势——战略协同、财务和人力资本以及专注的执行力——这使它们成为采用生成式人工智能的首选对象。私募股权公司为其投资组合公司设定了明确的目标和时间表,专注于在特定投资期限(通常为五年)内创造价值,这使得它们能够果断采取行动,优先考虑并实施生成式人工智能应用。获得私募股权支持的公司还可以获得生成式人工智能项目所需的财务和人力资本,使这些公司能够在预期增长的情况下大力投资于领导团队和顾问团队建设。因此,这些企业往往更愿意基于高回报的潜力而承担经过深思熟虑的风险。
中型企业利用生成式人工智能的策略
相较于大型企业,中型企业在采用生成式人工智能方面可能有一些结构性优势,但这并不能保证成功。以下列举了当前中型企业可采取的五大战略举措,旨在提升其成功运用生成式人工智能并实现价值创造的可能性。
1. 构建可扩展且灵活的生成式人工智能技术栈:投资可扩展的人工智能即服务平台,这些平台能够随着公司的发展而扩展,而无需大量额外投资。
2.转向“重塑”和“发明”: 除了部署生成式人工智能来逐步改进现有流程外,还要重新思考业务模式以及如何重新设计整个功能。波士顿咨询公司最近的一项调查发现,处于人工智能应用前沿的公司在核心业务功能中部署人工智能和生成式人工智能所获得的价值占比高达近三分之二(62%),其余三分之一(38%)则来自更多的外围支持功能。由此得出的启示显而易见:选择能够重新设计核心功能的深层应用程序,并优先考虑那些利用独特、专有数据来创建护城河的应用程序。
3. 关注能与生成式人工智能相辅相成的因素,而不仅仅是技术本身:正如Evolution Ltd最近发布的一份白皮书所指出的那样,人们对生成式人工智能感到失望的一大关键原因在于过于强调技术本身,而对上游(数据工程和专有数据)和下游(将生成式人工智能整合到战略决策中并创建学习和实验循环)的关注太少。
4. 建立清晰的治理和领导机制:要在生成式人工智能方面取得成功,公司领导层必须做出坚定承诺,构建有利于高效决策的治理结构,并优先考虑中期投资,而不仅仅是即时回报。
5. 增强员工能力:利用生成式人工智能增强员工技能,使他们能够完成超出当前能力范围的任务。
生成式人工智能:中型企业的“最佳时机”?
中型企业过去被认为规模太小,如今可能“恰好”适合充分利用当今的生成式人工智能。然而,要把握住这一机遇,它们需要制定明确的战略,并密切关注生成式人工智能能够发挥作用的领域——不只是降低成本,还要创造收入和价值。那些能够始终专注于有效推进生成式人工智能战略实施的企业会发现,人工智能革命并非只是行业巨头或灵活的初创企业的专属,而是包容性的变革浪潮,中型企业是搭乘“革命专列”的理想之选。(财富中文网)
弗朗索瓦·坎德隆(François Candelon)是私募股权公司Seven2的合伙人,曾任波士顿咨询公司亨德森研究所(BCG Henderson Institute)全球主管。
迈克尔·G·雅各比德斯(Michael G. Jacobides)是伦敦商学院唐纳德·戈登爵士创业与创新教授,同时担任波士顿咨询公司亨德森研究所学术顾问和Evolution Ltd.首席顾问。
米纳尔·波尔(Meenal Pore)是波士顿咨询公司的负责人,同时也是波士顿咨询公司亨德森研究所的代表人物。
列昂尼德·茹科夫(Leonid Zhukov)现任波士顿咨询公司全球人工智能研究所所长,并兼任BCG.X人工智能与数据科学副总裁。
本专栏文章中所提及的部分公司是作者所在企业过去或现在的客户。
译者:中慧言-王芳
Despite generative AI’s remarkable advances in recent years, adoption of the technology remains largely confined to the same large corporations that have historically led the way in deploying emerging technologies. But GenAI is evolving and so, too, is the company profile best suited to extract value from it. Increasingly, it is mid-sized companies that possess the right balance of resources and agility to accelerate adoption, drive meaningful outcomes, and reap the benefits of GenAI as the technology matures.
Large enterprises currently dominate GenAI adoption. A 2024 BCG survey found that 46% of large companies reported mid-to-high levels of GenAI maturity, compared to just 28% of mid-sized firms. With greater access to talent, data, and capital, larger players have had an edge in taking on traditional, resource-intensive AI initiatives.
But that advantage appears to be fading. Recent research from the London Business School (LBS), the Institute of Directors (IoD), and Evolution Ltd suggests that mid-sized companies—particularly ambitious, growth-focused firms such as those backed by private equity—are well placed to overcome adoption barriers. Mid-sized firms are more agile than large companies, a key attribute as GenAI has become more accessible, making these companies prime candidates to leverage the technology and unlock their potential.
On the whole, while such firms are still behind, they may be poised to rebound. Research by Oxford Economics found that only a quarter of mid-sized companies surveyed had adopted AI in 2023 but 51% were planning to adopt AI in 2024; the adopters were expecting it to improve their outlook, specifically in new products and services (43%) and marketing and sales (48%).
Organizational scale: a double-edged sword
Until recently, it was (very) large companies that benefited most from GenAI, as the advantages of scale outweighed the challenges of organizational complexity that accompany size. Yet as technology evolves, large firms find themselves slow to adjust. Extensive layers of management, entrenched processes, and siloed operations can slow down the adoption of fast-evolving technologies like GenAI.
In large corporations, GenAI implementations can suffer from “death by a thousand pilots,” in which individual teams or functions develop proof-of-concept products and tools yet do not manage to scale them due to the enterprise complexity and lack of clear governance. As a result, large companies frequently struggle to fully realize the potential of new tools despite extensive investment in digital transformation efforts.
Mid-sized firms, by contrast, can benefit from leaner structures that allow for quicker decision-making and implementation, given the right leadership and governance. Their agility, when combined with the right strategy, enables them to adapt more quickly to new developments in the technology and more easily operationalize GenAI. (Mid-sized firms here refers to companies with revenues between $50 million to $1 billion, and although the precise definition will vary from country to country, this broadly refers to companies that are still small enough to have relatively simple operations and remain agile.)
While the benefits of size and scale provide once-decisive advantages in access to specialized talent and capital-intensive infrastructure, the evolution of GenAI as a technology—particularly the development of GenAI as a service, the emergence of streamlined platforms, and growth of customizable models—is creating a more level playing field between mid-sized and large firms.
GenAI providers, for instance, are significantly reducing the need for up-front investment and extensive IT capabilities, by offering models and infrastructure as a service. Streamlined platform solutions like Google Vertex AI and Snowflake also simplify the AI ecosystem, providing integrated tools for data management, model customization and deployment, all of which lower technical barriers and accelerate time-to-value.
The advance of customizable models through technologies like retrieval-augmented generation (RAG), meanwhile, allows mid-sized firms to leverage their proprietary data effectively without an army of in-house data scientists. Much of the coding needed to build traditional AI has been replaced with natural language prompt engineering to create GenAI-powered tools tailored to the company’s content, expertise, and workflows.
In addition, updates to existing software platforms including ERPs and CRMs are incorporating AI features, giving easy access to AI functionality on the existing tech stack. Mid-sized companies are well placed to adopt these rapidly, given they generally have less complex and less customized instances of software, so integrating new releases is simpler and faster than for larger companies.
Taking GenAI from adoption to value creation
Beyond adoption, mid-sized companies are well positioned to create value from GenAI, as it may help them tackle the operational constraints that often hold them back. Mid-sized firms often struggle to attract specialized talent, such as data scientists, and do not have the scale to make it economically viable to hire a full-time position. GenAI tools can expand the capabilities of existing staff, as demonstrated by a recent BCG experiment where management consultants were each asked to complete three basic data-science tasks outside their core consulting capabilities: data cleaning, predictive analytics, and statistical understanding.
Using GenAI to perform the tasks immediately expanded the consultants’ aptitude beyond their current abilities. These augmented participants showed a 13- to 49-percentage-point improvement over those working without GenAI and came within 12 to 17 percentage points of the benchmark for data scientists. Function- or role-specific tools are now entering the market and enabling companies to further expand the capabilities of existing employees. Sisense, for example, enables companies to build semantic data models without coding that users can then query through natural language queries, enabling managers to incorporate data-driven insights into their decision making without the need for data analysts or data scientists.
Another constraint often found at smaller companies is a lack of sufficient proprietary data to create differentiation. The recent study by LBS, IoD and Evolution Ltd. found just 56% of smaller firms with annual revenues of £10 million to £50 million stated they believe that proprietary knowledge is somewhat or extremely important to their business, compared with 72% of mid-sized companies with revenues over £50 million. Large companies, on the other hand, are already using traditional AI to extract value from proprietary data, having invested in cleaning and curating datasets.
Mid-sized firms, however, often have a wealth of unstructured data—from which they’ve struggled to extract value. A mid-sized company, for example, may have handbooks for customer service agents outlining product details and troubleshooting tips, along with transcripts of real customer support calls. With GenAI, such a firm could now unlock those insights without needing to hire a team of data scientists, using company data to make new connections, and creating and disseminating highly tailored organizational knowledge in real time. The result is improved customer service at a reduced cost—something that these companies would previously not have had the resources, capabilities or infrastructure to do.
Mid-sized companies backed by private equity firms have additional operational strengths—strategic alignment, financial and human capital, and focused implementation—that make them prime candidates for GenAI adoption. PE firms’ clear objectives and timelines for their portfolio companies, focusing on value creation within specific investment horizons (usually five years), enable decisive action to prioritize and implement GenAI applications. Companies backed by PE can also access the necessary financial and human capital for GenAI projects, giving these companies the capacity to invest heavily in leadership and advisory teams in anticipation of growth. As a result, they are often more willing to take calculated risks based on potential for high returns.
Strategies for mid-sized companies to harness GenAI
Mid-sized companies may now have some structural advantages for GenAI adoption compared to larger players, but that doesn’t guarantee success. Here are five strategic steps they can take right now to increase their chances of successful GenAI adoption on the road to value creation.
1. Build a scalable and flexible GenAI stack: Invest in scalable AI-as-a-service platforms that can grow with the company without significant additional investment.
2. Move to ‘reshape’ and ‘invent’: Move beyond deploying GenAI for incremental improvements to current processes, and rethink your business model and how you can reengineer entire functions. A recent BCG survey found that the companies at the forefront of AI adoption derive nearly two-thirds (62%) of the value they get deploying AI and GenAI in core business functions, with the remaining third (38%) coming from more peripheral support functions. The takeaway is clear: Go for deep applications that reengineer core functions and prioritize those that leverage unique, proprietary data to create a moat.
3. Look at what complements GenAI, not just the technology: As a recent Evolution Ltd white paper suggests, a key reason for disappointment with GenAI is an overemphasis on the technology itself with too little attention paid to what lies upstream—data engineering and proprietary data—and downstream—integrating GenAI into strategic decision-making and creating learning and experimentation loops.
4. Establish clear governance and leadership: Success with GenAI requires a strong commitment from a company’s leadership to implement governance structures that facilitate efficient decision-making and prioritize investment for the mid-term, not just immediate returns.
5. Enhance workforce capabilities: Use GenAI to augment employee skills, enabling them to perform tasks beyond their current capabilities.
GenAI: The ‘just right’ moment for mid-sized companies?
Mid-scale companies, once considered too small, may be “just right” to make the most out of today’s GenAI. To do so, however, they need a clear strategy and a tight focus on where GenAI can make a difference—not just reducing costs, but generating revenue and value. Those that are able to stay laser-focused on effective implementation will find the AI revolution is not just for the industry incumbents or nimble startups—it can be an inclusive wave that mid-sized companies are ideally suited to ride.
François Candelon is a partner at private equity firm Seven2 and the former global director of the BCG Henderson Institute.
Michael G. Jacobides is the Sir Donald Gordon Professor of Entrepreneurship and Innovation at London Business School, academic advisor at the BCG Henderson Institute, and the lead advisor of Evolution Ltd.
Meenal Pore is a principal at the Boston Consulting Group and an ambassador at the BCG Henderson Institute.
Leonid Zhukov is the director of the BCG Global A.I. Institute and vice president of AI & Data Science at BCG.X.
Some of the companies mentioned in this column are past or present clients of the authors’ employers.